Three observations from the kick-off of a new consensus study by the National Academy of Sciences on measuring U.S. economic inequality
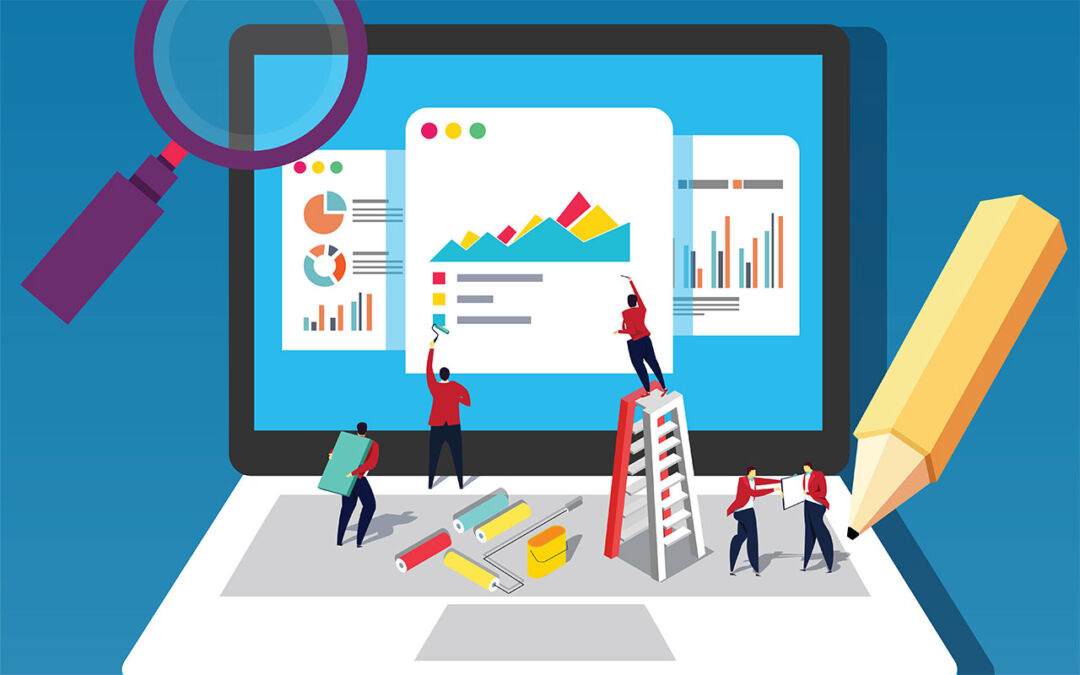
A new National Academy of Sciences panel convened publicly for the first time this week to discuss how statistical agencies should unify and improve the measurement of inequality in income, wealth, and consumption in the United States. This consensus study will debate how these concepts should be measured, how to harmonize these connected concepts, to what degree it should be possible to disaggregate them by geography and demographic characteristics, how they could be constructed using current data or data that are yet to be created, and more.
Equitable Growth’s Jonathan Fisher offered a six-point wish list before the event that covers some of the possibilities discussed during the first convening of the new NAS panel. But this is just the beginning of the panel’s work, which should wrap up sometime in 2023.
The first public meeting featured presentations by three prominent economists. Angus Deaton is a Princeton University professor and Nobel prize winning co-author of Deaths of Despair alongside Anne Case, the Alexander Stewart 1886 professor of economics and public affairs, emeritus at Princeton University. Emmanuel Saez is an economist at the University of California, Berkeley, a professor, a John Bates Clark Medal winner, and a MacArthur Genius fellowship winner. Raj Chetty is a Harvard University professor and also a John Bates Clark Medal winner and MacArthur fellow winner who has transformed empirical economics with his big data research.
The three economists presented alongside representatives from U.S. statistical agencies, who spoke on their current distributional data projects. The first meeting highlighted three debates that will likely be central to the panel’s work.
How much guesswork is acceptable?
The meeting started out on a technical note, with Deaton expressing hesitancy over what we might broadly call statistical modeling to create government statistics, which includes techniques such as imputation and model-assisted estimation. He pointed specifically to a field of research known as the Distributional National Income Accounts, popularized by economists Thomas Piketty at the Paris School of Economics and UC Berkeley’s Saez and Gabriel Zucman. Distributional accounts research seeks to take an aggregate income concept, such as Gross Domestic Product, National Income, or Personal Income, and distribute it among persons or households in an economy.
A common step in the production of such accounts is called “scaling.” Researchers start with a survey or administrative data source that provides information on incomes, among them IRS tax returns or the Current Population Survey. They add up all income in their base data to get an aggregate for the economy. But, for known and unknown reasons, these aggregate totals are almost always smaller than the total recorded in national accounts. To reconcile them, researchers simply scale up, inflating the wages or business income of each person in their data by a set amount so the new aggregate in the dataset matches what is reported in the national accounts.
This is the approach the U.S. Bureau of Economic Analysis takes in its Distribution of Personal Income prototype data series. Deaton is rightly concerned that scaling is a bit of a Band-Aid that ignores what the underlying causes of these aggregate discrepancies might be. Better source data, using a combination of administrative data and survey data, should be leveraged to try to explain why these gaps exist. It may be difficult, however, to eliminate these gaps altogether.
Deaton also expressed skepticism about other assumptions that are commonly made in the construction of distributional national accounts. These assumptions should be examined, but Deaton is overly pessimistic. There are several reasons we should be willing to use statistical modeling to construct official statistics.
Perhaps the most convincing argument is that these statistical Band-Aids are already being employed in many of our federal statistics and have been since the federal government first started recording them. A significant fraction of Gross Domestic Product and other national account aggregates are already being imputed because there is no other basis for estimating them. Early estimates of these metrics include more imputed information, as they are published before all data are received and they are revised several times.
Modeling can make incredibly useful contributions to our national statistics. Consider the U.S. Bureau of Labor Statistics’ recent experimental release of estimates that extend the Job Openings and Labor Turnover Survey to metropolitan statistical areas. These estimates are not possible, given the existing JOLTS sample sizes. The agency’s experimental release uses a modeling technique known as small area estimation to create estimates for these small geographical areas. This allows it to report statistics for small geographical areas without adding expensive oversamples to the survey itself. That’s incredibly valuable for statistical agencies, where resources are often tight.
Nor should we let a fear of imputation and modeling stand in the way of reporting important concepts. The underlying logic of Distributional National Accounts is compelling: Integrating microdata into our economic aggregates allows the statistical agencies to fully account for economic income and answer questions about who is benefitting from economic growth. Knowing the answers to those questions is a compelling public good.
No economic metric is without error. The role of our statistical agencies is to balance the utility of a measurement for informing the public and steering policy against the amount of error that might be present. Statistical agencies should continue to study model-assisted estimations because, in many cases, they are the only options for constructing a metric. Moreover, this research can yield valuable insights into sources of error in both our micro- and macro-data sources.
How ambitious should the federal government be?
Closely related to the debate over imputation is the question of how ambitious federal agencies should be. In the second half of the meeting, we heard from a number of statistical agency economists about projects they are working on that will expand the U.S. statistical capacity considerably. I have written extensively about BEA’s landmark distributing personal income product, presented by BEA’s Dennis Fixler, the only product from a statistical agency that tries to measure inequality using a comprehensive definition of income.
Jonathan Rothbaum of the U.S. Census Bureau presented his team’s efforts to link administrative and survey data to create more comprehensive databases of economic outcomes. And statisticians from the U.S. Bureau of Labor Statistics and Statistics of Income, the IRS’s statistical agency, likewise showcased impressive projects to improve the measurement of consumption and income.
There is always more to be done, and the meeting highlighted some areas of work that would be quite new to statistical agencies. Harvard’s Chetty, for example, advocated for a national metric on mobility. This may be slightly outside the panel’s purview, but the panel’s work might make such a metric more feasible.
This metric would probably be controversial, however. There are relatively few longitudinal datasets that allow for direct observation of mobility in the United States. Chetty instead uses cross-sectional data and some clever assumptions to generate estimates of mobility over time. Jonathan Davis and Bhashkkar Mazumder at the Federal Reserve Bank of Chicago do use a longitudinal dataset—the National Longitudinal Survey of Youth—and find similar trends, providing some support for Chetty’s methods.
Mobility may also strike some economists as outside the traditional purview of federal statistics. Nonetheless, I agree with Chetty, who remarked that providing official measures of mobility would “be incredibly valuable for the public discourse.” U.S. residents should know if the nation’s economy is putting the American Dream further and further out of reach, as Chetty’s research demonstrates. That is valuable information that will make voters more informed and more able to hold their elected officials to account. It is equally important for elected officials themselves, who hopefully want to strengthen one of the nation’s foundational values.
Measuring consumption is hard
Consumption was, in some ways, the least discussed of the three measurement concepts this panel is tackling. The Bureau of Labor Statistics presented briefly on some of their work to modernize the Consumer Expenditure Survey, but most other presenters mentioned consumption only fleetingly if at all. Princeton’s Deaton suggested that focusing on wealth was paramount. Chetty advocated for mobility, which generally means income or wealth, although he did mention that it would be useful to measure mobility using consumption. UC Berkeley’s Saez largely focused on wealth and income.
There is an active debate about whether consumption inequality is more or less important than wealth and income inequality. In some ways, that debate is waiting for better data, because measuring consumption is very difficult, and most economists would probably agree that of these three concepts, we know the least about consumption. The Consumer Expenditure Survey has known problems related to how people answer questions that complicate interpretation.
The cutting edge of consumption research in academia uses transaction data from banks and credit card companies to track what people are spending money on. Sources such as Earnest Research make it possible for Equitable Growth grantee Jacob Robbins of the University of Illinois at Chicago, for instance, to track how consumption responded to a wave of retail restrictions during the pandemic.
Unfortunately, there are numerous obstacles to government agencies using these kinds of data in the production of official statistics. These datasets are not representative of the entire U.S. population since they track only credit card users and individuals with bank accounts. They don’t include cash transactions and can miss transactions if a household uses more than one credit card provider. The datasets are expensive, a problem for cash-strapped federal agencies. And working out terms of use with banks and credit card firms can be a significant challenge.
Finally, there is no guarantee that a private dataset will be consistent over time. Either the data provider or the underlying producer of the data (banks and credit card companies) could make changes to data collection that break time series. Consistent time series is one of the greatest achievements of the federal statistical system, giving researchers treats such as an uninterrupted 74-year data series of unemployment rates measured in nearly the same way in every period.
Ultimately, government statistics might rely on a mix of survey and transaction data, or perhaps significant changes to how the Consumption Expenditure Survey is administered to improve respondent accuracy and develop a more complete set of U.S. consumption data to measure this aspect of U.S. economic inequality.
In Conversation with Jonathan Fisher
December 16, 2021
Simplified distributional national accounts
January 28, 2019
The work ahead
The National Academy of Sciences assembled an outstanding panel of scholars to consider these issues. Equitable Growth is especially proud that there are seven Equitable Growth grantees, a member of Equitable Growth’s Steering Committee, and three current or former members of our Research Advisory Board on the panel.
The work of this panel is incredibly important. As several speakers noted, inequality in the United States increasingly means that the rich and poor live dramatically different lives. This panel, and the work of our federal statistical agencies, will lead to a better understanding of inequality that informs U.S. residents and provides actionable information for policymakers.