How the new Global Repository of Income Dynamics database sheds light on income inequality and economic mobility
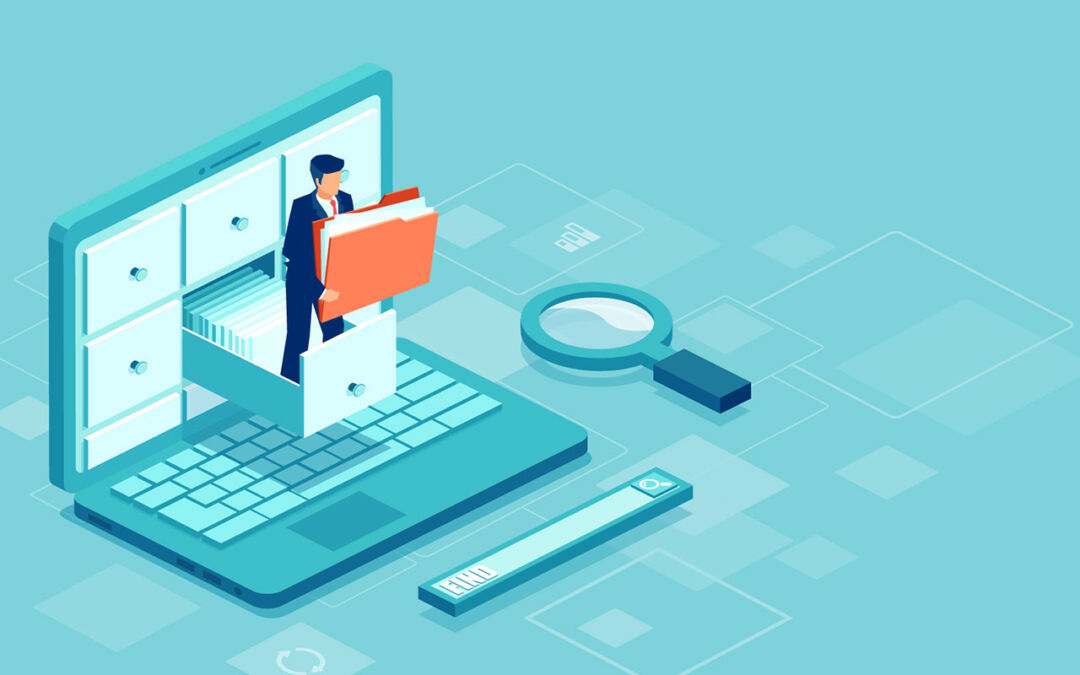
Economists have long known that a rigorous understanding of inequality is only possible with high-quality data. To this end, developments in economic models since the 1970s have helped advance our understanding of the distribution of earnings, as well as the dynamics of earnings—or how the income of an individual evolves over time. Nevertheless, important gaps in the research literature remain, most notably regarding subpopulations and income dynamics across time.
The Global Repository of Income Dynamics, or GRID—a new open-access, cross-country database that contains a wide range of micro statistics on income inequality, income dynamics, and mobility—helps to fill these research gaps. In a recent working paper published by Equitable Growth, the leaders of the GRID project—Fatih Guvenen of University of Minnesota, Luigi Pistaferri of Stanford University, and Giovanni L. Violante of Princeton University—introduce GRID as part of a recent special issue of Quantitative Economics and present some top-level trends in global income inequality and income dynamics from the GRID statistics. These trends only begin to scratch the surface, insofar as the database provides hundreds of thousands of summary statistics for a broad range of income dynamics.
The authors note there are four key features of GRID: It is longitudinal, administrative, granular, and harmonized. Taken as a whole, these features comprise a unique dataset that enables researchers to make cross-country comparisons more efficiently and provides rich administrative microdata that would otherwise be prohibitively costly or infeasible to collect and analyze. The four features also provide their own specific advantages.
First, GRID being longitudinal means that researchers can study the dynamics of an individual’s income over time, rather than static snapshots of distributions, as is the case with cross-sectional inequality measures. Longitudinal statistics are crucial for welfare analyses and designing social insurance programs. For instance, the authors cite a common disadvantage of a cross-sectional snapshot: the inability to draw welfare inferences from a constant poverty rate. A constant 10 percent poverty rate across two subsequent years is compatible with 10 percent of the population being permanently poor or with the entire population facing a 10 percent chance every year of falling into poverty. With cross-sectional data, the two cases are indistinguishable, whereas with longitudinal data, one can follow the fortunes of people over time.
Second, GRID’s administrative data has advantages over survey data for research on income inequality and income dynamics. Since U.S. administrative data are collected from Social Security records or similar retirement income programs in other nations, as well as from general tax records, the common disadvantages of survey data are avoided, such as sample attrition, smaller sample sizes, and measurement errors. To be sure, the authors note administrative data have its own disadvantages, such as cases in which the informal sector is large in certain countries. Nevertheless, the authors also note that in these cases, survey data can be used to supplement analyses, as was done by economist Daniela Puggioni of the Bank of Mexico and co-authors in their study of the evolution of income inequality in Mexico.
2023 Request for Proposals
November 9, 2022
Third, GRID is granular, meaning it provides micro statistics on income inequality, income fluctuations, and economic mobility for finely defined subpopulations. Computing disaggregated statistics is especially important because it is well-documented that different groups do not experience income inequality and macroeconomic dynamics uniformly. With disaggregated data, researchers can examine what geographic regions are being left behind, whether persistent systemic race and gender divides are narrowing, how an economy is performing for old and young workers alike, and much more.
Lastly, GRID is harmonized, meaning the data from different sources are combined so they can be viewed in a comparable way. Specifically, all statistics for all countries are produced by one unique master code in GRID, which ensures that a long list of small, but potentially critical, steps are carried out the same way in each country. This allows researchers to make cross-country comparisons efficiently and makes adding additional countries to GRID easier in the future.
The research that GRID helps open up is extensive. Not only can these statistics be useful in a wide range of inequality research, as the breadth of topics in the special issue of Quantitative Economics suggests, but they can also help inform other kinds of quantitative analyses whose structural models rely on income dynamics as a key input. As research on economic inequality trends continues to develop, it is imperative for this type of high-quality data to be accessible so researchers can advance new research questions and deepen our understanding of inequality.