Exploring the impact of automation and new technologies on the future of U.S. workers and their families
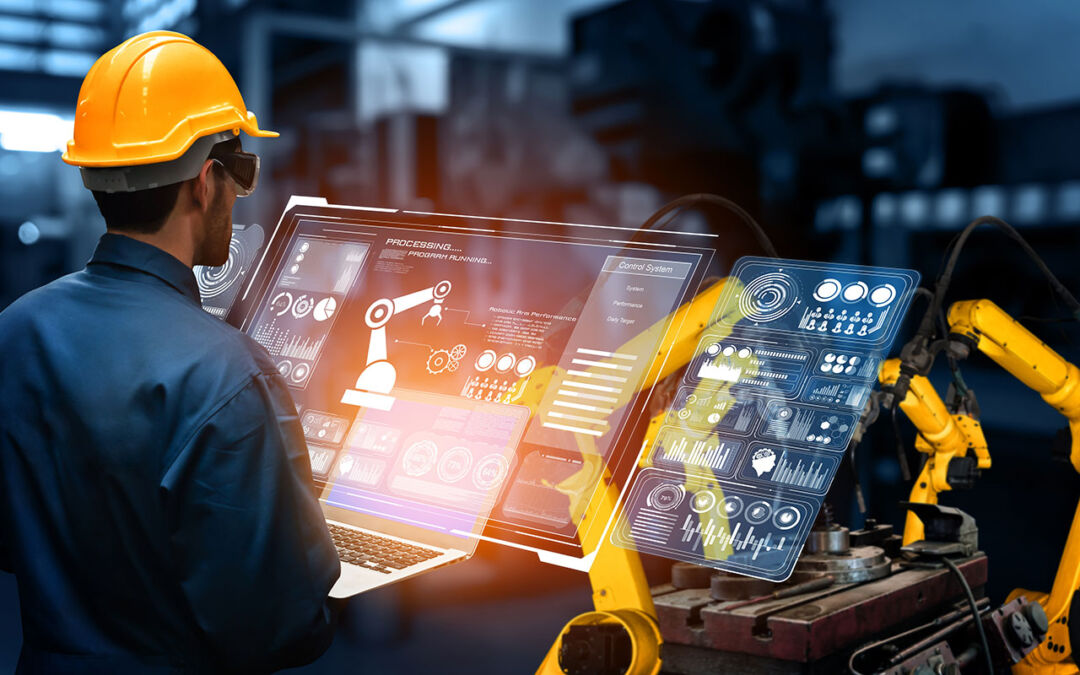
Overview
As technological advances seemingly reshape every aspect of how U.S. workers and their families live and work, a persistent question in research and policy discussions is how these advances will affect employment and the U.S. labor market in the years and decades to come. Employers could use automation and other advanced technologies to displace human labor or augment it to different degrees, replacing human labor or changing the nature of jobs without becoming a future without workers.
Ultimately, many factors affect how employers integrate technology into work, including the policy landscape and economic incentives. Likewise, many factors affect what impacts technology will have on the U.S. labor market, on working conditions, and on worker power. There remains more work to be done to understand how policymakers can shape the future of the U.S. labor market to ensure gains from technological integration can foster broadly shared growth.
This issue brief will first examine the factors shaping employers’ use of new technologies in workplaces, which is often driven by factors other than clear-cut efficiency and productivity gains. Then, it will look at the expanding role of automation technologies in workplaces and what that means for workers. The issue brief then closes with a look at how employers’ use of automation, artificial intelligence, and other advanced technologies could have different impacts on workers and society, and even improve working conditions and worker power.
How policy shapes employer decisions around the implementation of automation technologies
Many studies attempt to quantify how exposed different kinds of jobs are to technologies such as automation, computerization, or artificial intelligence. These predictions are often based on whether physical or cognitive tasks are “routine” and can be replicated by robots, digital processes, or increasingly sophisticated prediction algorithms. For instance, one commonly cited 2013 report from the University of Oxford suggested that almost half—47 percent—of jobs in the United States were at “high risk” of automation in the near future, “perhaps over the next decade or two.”
Researchers often estimate these possible outcomes by using data on tasks within occupations, as categorized by the Occupational Information Network, or O*NET, database. They use this database to label different tasks as more or less automatable or otherwise vulnerable to technological displacement, and then examine levels or trends within and across occupations over various time horizons.
Much more often than not, the focus on how exposed jobs are to new technologies may carry with it the implicit assumption that automation provides an incontrovertible business advantage—that automation will always produce an equivalent product, or even a superior one, and at a lower cost—and that displacement of human workers is ultimately inevitable. These assumptions are tied to what data journalist Meredith Broussard describes as technochauvinism, or “the belief that technology is always the solution.” Yet in many cases, the role of technology in these scenarios is not to produce a higher-quality product or service, or to increase efficiency. Rather, automation—the replacement of human labor with machines—becomes an end in itself.
As economists Daron Acemoglu at the Massachusetts Institute of Technology and Pascual Restrepo at Boston University discuss in a 2019 paper, the development of technologies that replace rather than complement humans is ultimately a choice. They posit that over the past 20 years in particular, automation technologies—those designed to replace human labor completely—were developed relatively faster, compared to technologies that complement human labor or create new labor-intensive tasks.
Acemoglu and Restrepo write that “our results suggest that it is the combination of adverse shifts in the task content of production—driven by accelerated automation and decelerating reinstatement—and weak productivity growth that accounts for the sluggish growth of labor demand over the last three decades and especially since 2000.”
The automation of human labor is not new, and it has had varying effects on U.S. employment and the U.S. labor market. For instance, a working paper examining the effects of electrification on U.S. manufacturing from 1890 to 1940 shows that electrification drove significant and long-lasting labor productivity gains, but employment effects were mixed. The researchers find that employment grew only in counties with small firms, but not in counties with large firms. They find the types of jobs changed, too. Within manufacturing, more complex craftsman occupations declined, and more routine production jobs rose. Within office work, routine clerical jobs declined, and professional and technical occupations increased.
Employment effects can also change over time. A working paper by economists James Feigenbaum at Boston University and Daniel P. Gross at Duke University on AT&T’s adoption of mechanical switching technology between 1920 and 1940 finds that the then-current telephone operators not only lost their jobs due to this automation, but also were more likely to be in lower-paid occupations or to have left the labor force entirely a decade later. But the two researchers also find that for new cohorts of workers who otherwise may have entered into this profession, “the decline in demand for operators was counteracted by growth in both middle-skill jobs like secretarial work and lower-wage service jobs, which absorbed future generations.”
Is today’s technological moment different? And if so, why? Researchers have come to different conclusions on this point, but Acemoglu and Restrepo’s analysis suggests that in recent decades, the labor-saving shifts from automation are no longer offset by new task creation or other impacts that increase demand for labor elsewhere. In addition, and in contrast to previous eras of technological change, they find that this accelerating shift to automation has not been accompanied by a similarly large rise in productivity.
Instead, employers have displaced workers with “so-so” technologies—such as automated customer service options or self-checkout kiosks—that provide a similar, or even inferior, service, compared to the human labor they displaced, and deliver only minor savings to the employers, if any. In Redesigning AI, Acemoglu writes that the current path of innovation in automation and artificial intelligence harms not only the displaced workers but also society as a whole, both through these labor market effects and through other negative externalities, such as the focus on AI-driven mass surveillance technologies.
This kind of research into the future of work provides further evidence that employer decisions around how to implement new technologies and their broader effects on individual workers and labor markets are not predetermined, but rather are intertwined with the policy, regulatory, and economic environment in which these jobs exist. Acemoglu and Restrepo, in their analysis, posit that this trend to “excessive” automation may be due to more structural factors that incentivize automation, such as a system that taxes capital such as machines and software at a much lower rate than labor.
The ‘human infrastructure’ underlying automation
Another complication in discussions of automation is that employers often use technology not simply to replace human input, but also to reorganize work and shift costs and risks away from their firms. In a report for the nonprofit research organization Data & Society, researchers Alexandra Mateescu and Madeleine Clare Elish explain that, crucially, employers often reconfigure work in ways in ways that render the labor invisible.
One example they discuss is the use of self-service kiosks in grocery stores, a common example of a labor-reducing technology. These kiosks, however, actually shift much of the labor from previously paid workers to current unpaid customers and yet, at the same time, create a new role for some employees to monitor, assist with, and troubleshoot the customer’s work.
As with many seemingly automated technologies, this “human infrastructure” is often invisible to customers, even though it is vital for the automation to function. Indeed, as Mateescu and Elish write, “the intelligence or autonomy of machines is made possible through the obfuscation of attendant human labor.”
This erasure is present in the very labor that makes artificial intelligence possible. Anthropologist Mary L. Gray and computational social scientist Siddharth Suri at Microsoft Research discuss this dynamic in their book, Ghost Work. They describe how workers all over the world perform “high-tech piecework” on Mechanical Turk and other distributed microwork platforms that invisibly shape and guide seemingly “autonomous” systems, often under poor working conditions and for extremely low pay.
Human workers play many roles in these seemingly automated processes. Some of them produce the training data that AI systems learn from, and others are often used as a final step of quality control. Some of these human workers may be even used to substitute for AI output altogether.
Associate professor at Georgetown Law Brishen Rogers explains that employers use data-driven technologies, including artificial intelligence and algorithmic management, both to de-skill work and undermine worker power, driving down job quality but also often resulting in a less efficient processes or lower-quality outputs. As a result, employers often use technology to reduce demand for cognitive skills and redistribute tasks among multiple, lower-paid workers. This happens despite claims that the use of advanced technologies necessarily leads to a higher demand for college-educated workers—and that the United States and other countries are now facing a “skills gap” and an inadequately educated workforce.
This redistribution and reorganization of work and tasks has had harmful effects on low-wage workers through declining job quality and economic mobility. And while many automation discussions focus on potential future impacts on production and service roles, automation processes are already widespread in many industries in the form of algorithmic management. In “Data and Algorithms at Work: The Case for Worker Technology Rights,” Annette Bernhardt, Lisa Kresge, and Reem Suleiman at the University of California, Berkeley Labor Center explain that employers’ use of data-driven systems can significantly harm workers through a variety of channels, including enabling discrimination and through health and safety harms from intense rates of work, along with losses of privacy and autonomy.
The use of data and algorithms can also harm workers and job quality through de-skilling and fissured work arrangements, leading directly and indirectly to job loss and lower wages. Algorithmic management and workplace surveillance are also used to reduce worker power, or to explicitly undermine employee attempts to organize.
Though automated processes are often seen as “neutral” or “data-driven,” algorithms actually codify management decisions—but in a way that makes them both inflexible and opaque. The introduction of AI and other technologies in management, in the words of Pauline Kim at the Washington University in St. Louis School of Law and Matthew T. Bodie at the Saint Louis University School of Law, “may bring employees a vortex of massive information collection, data vulnerability, and seemingly whimsical decision-making.”
Workers thus find themselves evaluated by a faceless system they can neither see nor challenge, as Aiha Nguyen describes in a recent report for Data & Society. And these workers often cannot appeal if they are punished or fired as a result. In addition to the more immediate harms to workers, Nguyen says this dynamic “creates a tremendous and invisible power imbalance between workers and companies.”
The use of technology in managing workers also introduces new avenues to deepen discrimination and differential harms throughout workplace processes. Though technology is often viewed as a way to reduce or eliminate discrimination, all technology is created by and within existing structures of power, as Princeton University African American studies professor Ruha Benjamin writes in her 2019 book, Race After Technology. “These tech advances are sold as morally superior because they purport to rise above human bias,” she writes, “even though they could not exist without data produced through histories of exclusion and discrimination.”
Women and the future of work in the United States
August 28, 2020
2022 Request for Proposals
November 10, 2021
For instance, many companies use technology to monitor, measure, and predict workers’ performance and output, as well as to prevent and audit security or safety concerns. Technologies that incorporate facial recognition, voice recognition, so-called emotional recognition, and other technologies related to worker biometrics can treat workers differently based on race, ethnicity, gender, age, disability status, or other factors.
Another avenue of algorithmic discrimination with potentially far-reaching impacts on labor markets and economic inequality is in hiring processes. Employers increasingly use digital hiring technologies and third-party platforms to recruit, filter, evaluate, and process large volumes of applications. Within the “black box” of an employers’ hiring process, the use of predictive and automated processes “both facilitates and obfuscates employment discrimination,” as described by Ifeoma Ajunwa, professor of law at the University of North Carolina School of Law.
Rather than treating algorithmic bias in hiring processes as a purely technical issue that can be addressed through better data and algorithms, Ajunwa explains that these technologies expose how the hiring process is already vulnerable to bias “due to an American legal tradition of deference to employers, especially allowing for such nebulous hiring criterion as ‘cultural fit.’”
As employers use digital hiring technologies to process large volumes of applications, these “nebulous” hiring criteria can even become systematically elevated or codified, such as requiring unnecessary degrees or disqualifying respondents based on personality tests. These often unexamined practices not only create and deepen discrimination and bias, but also advance credentialism and hinder effective job matches.
Automation technologies could improve working conditions and worker power, but the policy landscape needs to change
The process of technological innovation and job displacement has always been part of labor markets, but today, as in the past, this process does not have a “natural” or preordained endpoint. Researchers and policymakers can learn from the past and examine the policy and economic contexts of the present to help build future workplaces where workers and society at large benefit. Technological integration that allows for—and encourages—broadly shared growth can allow for improved social welfare, alongside increased productivity and innovation.
Equitable Growth’s 2022 Request for Proposals highlights some of the key research areas that can inform this path going forward. For instance, while automation’s presence is often more visible and more studied in the context of industrial robots and manufacturing, employers’ use of technology is affecting workers in a wide variety of ways, including through algorithmic management. Yet these types of uses—and their impacts—can be more difficult to observe and quantify, making them less visible in economic research and in policy debates.
New research in economics, sociology, and other fields can explore the various ways that automation and other technologies affect workers, from job quality to bargaining power, and ensure workers’ experiences are present in policy discussions. Research can also explore how employers make decisions about how to use technology in work processes, both historically and in the present, and how factors such as worker power and regulatory and policy landscapes affect these decisions.
Employers’ actions around integrating technology can, in turn, have broader effects on labor market dynamics and labor market flows, as well as the organization of work and the workplace. Future research can explore these impacts and whether employers are adopting technology to increase productivity or to entrench power dynamics. In short, automation technologies, better understood and supported by a shift in the policy landscape, could improve working conditions and worker power.