Accelerating research can help fill the AI knowledge gaps for U.S. policymakers
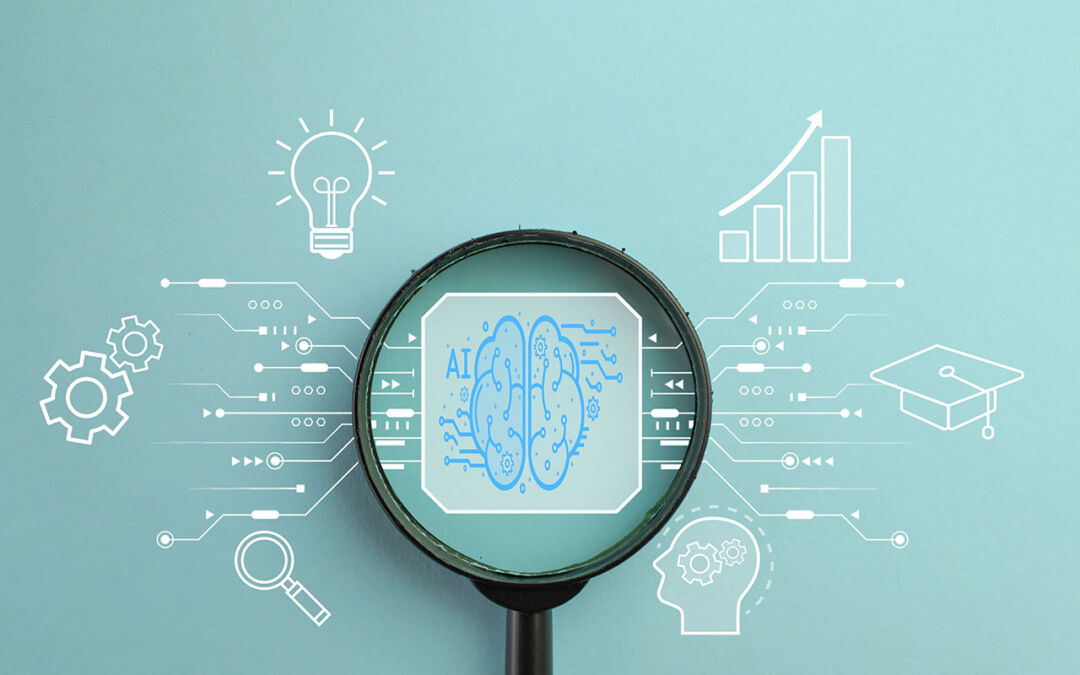
It is hard for policymakers to ignore today’s “AI arms race” and the public debate on the economic and social significance of generative artificial intelligence. The discourse is fueled in part by dramatic advances in the technology, as well as competing narratives on AI’s likely trajectory and how much its impact on the economy and society will ultimately matter.
Many companies espouse generative AI’s transformational benefits, while technology accountability groups warn of its dangers—both realized and existential, as well as ecological. What is more clear is that AI-driven technologies are being produced largely outside the purview of public authorities and academic research institutions.
Whether the current excitement over generative AI fades should developers and users discover that its drawbacks are insurmountable or governments and firms prove unwilling to overcome computing and data constraints, AI-enabled firms currently are poised to upend not only the tech industry in the United States but also wide swaths of our nation’s workforce across sectors. This uncertain landscape leaves policymakers unsure of how to effectively govern technology that is increasingly embedded into our digital and social infrastructure or, more fundamentally, how to reliably assess its current and future impact on the U.S. economy and society.
Past technologies are not a clear guide for forecasting the potential effects of generative AI. Yet many current policy challenges—with media disinformation, election security, and data privacy, to name a few—stem from the failure to thoughtfully regulate digital products emerging from a tech industry known for its “move fast and break things” mentality. So far, that industry, with its considerable resources, continues to dominate research and development on AI, leaving many critical questions either unaddressed or unanswered.
Indeed, massive investments in new AI applications, spurred by advanced computing power but dependent on larger tech companies’ capital and infrastructure, mean that only firms that already dominate the Fortune 100 boast the capacity to conduct the most powerful, novel research. In contrast, public research institutions face entry barriers in a resource-intensive space and are disadvantaged when competing with the tech sector for talent.
Furthermore, tech lobbyists that predominately represent larger companies are racing to fill in knowledge gaps in Washington. In the absence of comprehensive federal legislation, AI firms have largely been left to self-regulate and, at best, adopt voluntary standards.
That said, there are broad efforts afoot to understand how to structure regulatory guardrails and economic incentives that steer AI innovation toward the public good. Executive orders from 2019 and 2023 attempt to navigate the known bureaucratic challenges of tech governance at the federal level, though it is too early to evaluate their effectiveness and impacts. There also are efforts at the state and local levels that are mostly focused on privacy, surveillance, and transparency in AI development, as well as on the high-risk use of AI affecting consumers with regard to housing, health care, and employment.
Policymakers need a common knowledge base to govern complex, rapidly evolving, and widely applicable system AI systems, and they should not have to rely solely on evidence provided by the corporations commercializing this new technology. To help fill this knowledge gap, independent researchers must play a critical role in creating evidence-based frameworks and resources to guide current policy implementation and spur policy innovation.
This issue brief takes stock of what we already know about AI and its economic and social impacts to discern how researchers can best support policymakers in ensuring that the benefits of AI are broadly realized and shared. It will also detail the known and potential harms of AI to workers, communities, and the broader U.S. economy, so that those effects are mitigated efficiently and equitably. We close with a series of recommendations that call for policymakers to:
- Broaden the sources of independent research and analysis and not rely solely on dominant tech firms for information on AI
- Approach governance with both flexibility and sensitivity to specific sectors and uses of technology
- Advance policies that address underlying uncertainty and anxiety caused by economic inequalities that could be prolonged or exacerbated by AI
In these ways, leaders can embrace AI policymaking that promotes competitive and innovative markets, drives economic productivity, and supports workers while addressing the structural inequalities exacerbated by AI-driven technologies.
An emerging understanding of AI and its impacts
Underpinning an emerging base of knowledge on artificial intelligence, which has been around for years but only recently has made some notable advances, are definitions that continue to vary across fields and purposes and will need to be updated to reflect technological shifts. Our society’s understanding of AI is partly based on past technological impacts on the economy and projections of a theoretical future AI, compared to AI as it currently exists—AI that is neither “magical” nor “sentient.”
Today, AI systems are viewed by some as novel, distinct technologies that will become a general purpose technology, much like electricity, affecting all sectors of the U.S. economy. Opinions vary on the scale and speed of its development, as well as the nature and scope of its impacts on the U.S. economy and our society. “The actual state of the technology seems like the biggest source of uncertainty, rather than the effects of its most extreme form,” observes Daron Acemoglu, a Massachusetts Institute of Technology economist and an Equitable Growth Steering Committee member. Acemoglu argues that the effects of AI are not so different from past eras of technological change or even current ones, such as still-rising automation, when problems of inequality, stagnation, and unemployment were and continue to be as much a function of policy choices and power dynamics in the U.S. economy as they are of scientific progress.
In our view, a useful set of definitions come from Stanford University’s Human-Centered Artificial Intelligence Institute, which helps to distinguish between AI, components or specific types of AI, and terms sometimes used interchangeably with AI by nontechnical audiences.
We also can look to Executive Order 14110, issued in 2023, as a resource on AI across the federal government. This executive order:
- Defines artificial intelligence as a machine-based system that can, for a given set of machine- and human-based objectives, make predictions, recommendations, or decisions influencing real or virtual environments in an automated manner
- Defines an AI model as something that “implements AI technology and uses computational, statistical, or machine-learning techniques to produce outputs from a given set of inputs”
- Classifies generative AI as a class of AI models that emulate the structure and characteristics of input data to generate derived synthetic content, including images, videos, audio, text, and other digital content
Additionally, the White House Council of Economic Advisers’ 2024 report (on page 246) describes how data/inputs, algorithms, and computing power are used to “train” a predictive AI model that interacts with traditional automation in order to produce an action that emulates human-derived creativity and output. (See Figure 1.)
Figure 1
While our national data infrastructure must be modernized to be able to properly measure the state of AI and evaluate AI policies and programs, government agencies and academics have started to tackle how to reliably measure the actual state of AI diffusion in the economy.
In addition, economists have been calling for more analysis of the macroeconomic impacts of AI. The reason: AI-induced economic growth is likely to be heavily dependent on the rate at which that growth continues to produce new innovations rather than simply by virtue of ever-increasing computational or data resources.
As we navigate this transformative era, policymakers will need a working set of metrics for how to wholistically evaluate the impact of AI on the productivity of firms and workers, on the well-being of workers and communities, and on inequality, market competition, innovation, and other critical indicators. A central political and cultural challenge will be how to center worker and competition concerns in an area dominated by national security and financial interests.
Let’s now consider these key policy areas briefly in turn and the evidence gaps that policymakers face in each area.
Competition challenges in the face of AI
There is an economic upside for policymakers to take a proactive approach to regulating an upstart and strategic industry such as AI: Doing so can promote efficiency and consumer benefits, reduce costs of enforcement, and increase incentives for new firms to enter the AI marketplace. But this approach will be tricky to navigate.
Historically risk-averse antitrust and procurement bodies need to more closely scrutinize how AI adoption is changing the competition dynamics within and beyond digital markets. At the same time, there are geopolitical incentives for the United States to be a leader in AI, which makes it challenging for policymakers who see the merit in competition in the long term but do not want to be seen as putting restrictions on innovation, domestic market growth, and a competitive edge over other countries.
For policymakers to right-size their regulatory efforts, it is critical to understand the unique costs and barriers to the adoption of AI, why the AI sector is dominated by just a handful of major technology firms in the United States, and why it is particularly susceptible to “market failure” (antitrust parlance for allowing monopolies to form to the detriment of robust competition and consumers). Because of the new technology’s voracious need for computing power and data collection, the high costs of ensuring the quality of the inputs and outputs of AI products and services, and the limited supply of technical labor, large firms are more able to invest in producing and adopting AI models, while taking on certain risk and liabilities from investing in a novel technology.
This intense market concentration has, in turn, resulted in power imbalances and accusations of monopolistic behaviors, and antitrust authorities around the world have expressed concern with the scaling market power of dominant firms. Policymakers need new frameworks and tools, as well as more in-house and external technical expertise, to properly discern whether and how the well-documented negative consequences of reduced competition—including higher prices, higher inequality, and lower rates of innovation—apply in the AI context and if the current regulatory landscape can adequately address this challenge.
Currently, basic supply chain mapping of artificial intelligence is nonexistent, and a more nuanced understanding of the flow of capital and investments could provide insight into how markets are taking shape and where policymakers should focus their attention. Since traditional tools may be insufficient to meet the speed of technological progress and existing levels of concentration, researchers and policymakers also should consider the proposals for enhanced data disclosure, public investments and public-private partnerships in AI infrastructure and R&D, and digital taxes to address the unique tendency toward concentrated market power in digital markets.
Other questions that need to be broadly answered include:
- What regulatory or oversight mechanisms have worked in the technology sector and other industries that can be applied to the AI market?
- Do existing firms in the “tech stack” (or the various technologies needed to deploy AI) have too much market power? If yes, what are the implications for workers, innovation, and competition?
- What are the investment relationships or partnerships between firms in the AI space, and what are the implications for market structure and competition?
- What barriers to entry and network effects (or when the market value of a product or service increases based on the extent of its use) exist that could stifle innovation or competition in the AI market?
- What are the relationships between different markets in the tech stack, and what are the implications for market power and structure across various points in the AI supply chain?
AI is already reshaping the U.S. labor market
The growing use of artificial intelligence in the workplace has already had profound consequences for the U.S. labor market. Policymakers are grappling with how best to regulate AI-driven technologies that are used to surveil and manage workers, determine wages, and shape job search and hiring decisions—all of which have implications for data privacy, proprietary interests, safety and health, and workplace organizing, and can lead to bias, discrimination, and other harms. Meanwhile, businesses and researchers are exploring beneficial use cases of AI for workers in caregiving, weather and climate disaster forecasting, and supply chain management.
Researchers have documented how the adoption of AI replicates existing workplace inequalities and threatens workers’ bargaining power. Research also suggests that generative AI—which has been more rapidly adopted at work, compared to past technological changes such as the internet and the personal computer—can improve the productivity of less-skilled employees within an occupation or organization, and flatten firm hierarchies. These effects, at least in the short term, narrow the productivity gap while reducing inequality, without harming higher-skilled workers.
Frameworks for understanding the impact of technology on the labor market using historical data also are available. They include the evolution of economic thought on the impact of technological change in the labor market, the role of automation in workplaces, and how unions can moderate the impacts of automation. Several policy research and grassroots groups are actively engaged in collecting data and sharing policy resources for tech accountability and workplace protections. Yet there is limited nonindustry analysis on which jobs and demographics would be most exposed to AI deployment and how those impacts will complement the existing U.S. labor force and substitute AI for workers.
Then, there is the necessary work to be done in scenario planning. This includes updating social programs to offset the workforce implications of more disruptive AI and what kind of investments in human capital and workforce training would best serve a more AI-reliant U.S. economy, while also ensuring opportunity and security for those displaced by technological changes.
Policymakers will require a clear, easy metric of job quality for measuring AI’s impact and its potential role in the growth of “good jobs,” as well as a more sophisticated and innovative suite of tools to ensure worker safety and voice in the adoption of productivity-enhancing AI in the workplace. Questions that need to be broadly answered include:
- How are employers deploying artificial intelligence, and for what purposes? Are there notable gaps in employers’ understanding of AI technology that affect how they are using it, and what role do tech vendors play in addressing or not addressing any knowledge gaps?
- How do employees perceive the purpose of AI adoption, and do those views converge or diverge from employer perspectives? Can employers learn from employees that may already be engaging with AI tools?
- How and why will AI adoption vary across occupations, sectors, and geography?
- What institutions, including and beyond unions, are associated with successful mediation of how AI is being adopted and deployed?
- How will AI adoption impact bargaining power, health, safety, productivity, authority, job satisfaction, and other measures of worker well-being?
Conclusion and key takeaways for policymakers
AI is developing without the infrastructure to ensure the gains from its transformative growth are broadly shared. Already, serious harms and risks with AI technologies are growing and wide-ranging. While we are in the early days of understanding the myriad ways that artificial intelligence will change the U.S. economy and society, our nation is behind in investing in credible research and policy resources to help policymakers navigate this nascent, but fast-growing technology.
What’s more, the loss of the Chevron deference—the decades-long legal precedent arguing that government agencies could interpret and enforce congressionally enacted legislation, which the U.S. Supreme Court earlier this year struck down—means that there is an urgent need for more sophisticated technical knowledge within regulatory agencies. Without comprehensive federal AI legislation and public investments, tech and financial companies with a documented interest in consolidating both market and political power will have greater latitude to challenge new AI regulation and less incentive to invest in socially optimal products.
Policymakers will need new tools, metrics, and research to make both an evidence-backed and compelling case for AI oversight. They can do so in three broad ways.
First, policymakers should broaden the sources for research and analysis, and governments should not cede all the research incentives and opportunities to well-endowed tech firms. Economic analysis is crucial for understanding policy trade-offs and informing policy decisions, and academics can provide solutions to dynamic, complex political and economic challenges posed by AI technologies that are still evolving.
Government agencies should build and leverage relationships with academic institutions to support data building and knowledge sharing, and strategically steward research and development funding toward policy-relevant agendas and innovation that serves the public interest. Government also must continue to invest in the recruitment of technologists who can help modernize its infrastructure and better anticipate how the growing adoption of AI will reshape society.
Second, policymakers must resist a one-size-fits-all approach to AI governance, as cases involving the use of AI are varied and complex and will require different approaches depending on the sector and the technology in question. Some argue that the U.S. market-driven approach has fostered more digital innovation and growth in the tech sector, relative to the EU’s top-down regulatory approach.
Whatever the case, experimentation with policy tools at the federal level—procurement, program administration, and conditional funding for how AI is used in government—can model best practices for AI deployment, guide state- and local-level policy development, and popularize ideas that can turn into future legislation. A more balanced and flexible approach to regulation and government oversight should involve collaboration between government, industry, labor and civil society, and international partners to help address specific risks while allowing for continued and beneficial innovation.
Third, policies to govern AI should not only be reactive, but rather should also leverage this moment of existential concern to address underlying uncertainty and anxiety caused by economic inequalities. One recent survey shows the general public and workers are aware of and concerned about the deployment of AI and are acting on those attitudes. Another survey shows that an overwhelming majority of Americans believe that powerful technologies should be carefully regulated.
How policymakers handle AI in the next few years will inform the potential role of the government in steering technological development for the public interest in other promising, economically critical industries, such as advanced robotics and quantum computing. Policymakers should reject the false binary choice between innovation and equity, and act on a positive vision of AI that supports both productivity and workers, while addressing the structural inequalities exacerbated by AI-driven systems.
Did you find this content informative and engaging?
Get updates and stay in tune with U.S. economic inequality and growth!