Algorithmic wage discrimination requires policy solutions that enforce predictability and the U.S. spirit of equal pay for equal work
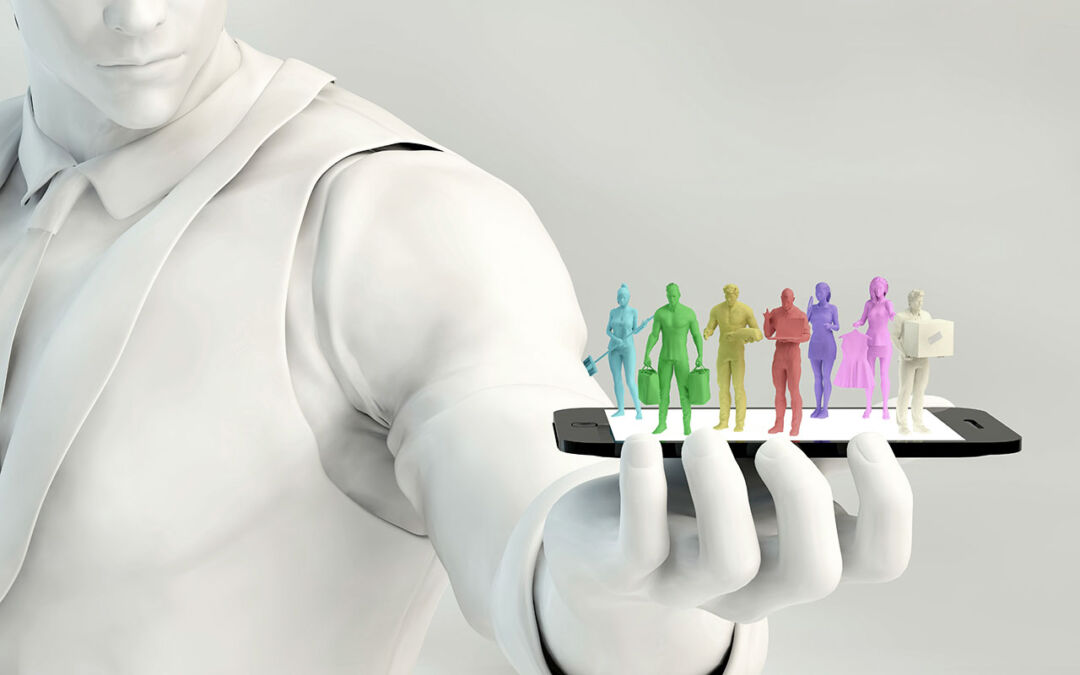
U.S. consumers generally believe that gig workers who labor for companies such as Uber Technologies Inc. and Lyft Inc. are free to set their own hours and thus able to determine broadly how much they will earn while ferrying passengers to and from their different destinations. Consumers of these services make a series of assumptions based on shared knowledge of how employment arrangements in the United States work or should work.
Riders probably assume, for example, that the longer gig workers labor at the wheel, the more they will make per hour, and that skills acquired over time mean that these workers can improve their hourly pay because, say, they can learn what areas and times are lucrative or how to game the algorithms.
But, as it turns out, these assumptions do not pan out. Gig work is far from flexible. Gig workers are highly “incentivized” through pay structures to work at specific times. Not working at those times or in the ways that Uber and Lyft want their contract drivers to work can result in them losing money, instead of earning it.
Using data extracted from drivers’ labor and then fed into machine-learning technologies, these companies can personalize base pay and the opportunities to raise base pay. This may sound like a rewards system for individual hard-working gig workers, but in fact because every gig driver is ostensibly an “independent contractor,” the two companies can drive down individual pay while maximizing their own corporate profits.
Counterintuitively, according to Uber’s own research, workers who labor for longer typically make less per hour. So even if drivers are working at the same time, with the same skills, in the same places, they may earn vastly different amounts.
Though economists and policymakers alike are used to discussing these dynamic algorithmic practices in terms of consumer pricing, these wage-setting practices have real and harmful effects on workers, too. Gig platform companies make a point of describing how their “surge pricing” makes good business sense for themselves and their customers. Yet their individual “surge payments” to their drivers—alongside any number of other wage products such as “bonuses” and “quests”(gig work-speak for complying with the companies’ rules to increase your base pay) results in unpredictable, variable, and personalized hourly pay that upends our legal and social expectations of “equal pay for equal work.”
Indeed, gig drivers often describe their experience of these algorithmic systems explicitly in terms of gambling. They are always hoping they will “hit the jackpot” with a high-paying trip while the app tries to trick them by giving out just enough rides to keep them on the road.
My new working paper, “On Algorithmic Wage Discrimination,” is the result of my almost decade-long ethnographic research on gig drivers in the San Francisco Bay Area. Among other things, I find that the digital, black-box structure through which wages are set among these workers results in unpredictable and variable pay, often changing from person to person and hour to hour.
Workers described the opaque, unpredictable, and variable pay practices to be fundamentally unfair and harmful. I argue that they are also fundamentally at odds with U.S. legal and cultural views of fairness at work. Digitally variable, personalized pay represents a historical rupture in how wages are determined, how work is coordinated, and how income is distributed between employees and employers arising from the data-driven logic of informational capitalism.
How should policymakers respond? I propose a non-waivable ban on the practice of algorithmic wage discrimination. This could include a ban on digitally determined pay per ride or on digitally personalized pay more broadly. Such a ban would put an end to the gamblification of work and the uncertainty of hourly wages that are endemic to the on-demand sector. And a ban also would disincentivize certain forms of data extraction and retention that may harm low-wage workers down the road, addressing the urgent privacy concerns that others have raised.
Similar to proposed bans on targeted advertising, which attempt to limit the use of personal data to make money from targeted ads, a peremptory ban on algorithmic wage discrimination might also disincentivize the growth of fissured workplaces under informational capitalism. If firms cannot use algorithmic gambling mechanisms to control worker behavior through variable pay systems, then they will have to find ways to maintain flexible workforces while paying their workforce predictable wages under a fair employment model.
This kind of ban is not without precedent. The spirit of a ban on algorithmic wage discrimination is embedded in both federal and state level antitrust laws, as I argue in my working paper. If workers are consumers of on-demand ride-hailing companies’ technology—as these companies claim—and not their employees, then digitalized variable pay in the on-demand economy violates the spirit of the 1936 Robinson-Patman Act, which was enacted to prohibit anti-competitive price discrimination.
Towards Justice, a non-profit legal organization, recently sued Uber and Lyft under California state antitrust laws, alleging violations of the state’s Cartright Act (the rough equivalent of the federal Clayton Antitrust Act) and California Business and Professions Codes that prevent secret commissions and other fraudulent practices. But enforcement actions such as this will take years and may not prevent these practices from migrating to other sectors. Hence, I propose a ban.
The precise limits of a proposed non-waivable ban need to be explored, and many questions remain in the statutory construction of such a ban and in its coverage, among them:
- Should such a prohibition be limited to companies with a controlling market share, as Fordham University School of Law’s Zephyr Teachout suggests?
- Should the ban only rule out digitalized variable pay between workers, but still allow a company to use algorithmic assessments to change how much it pays workers as long as those changes are applied to everyone?
- Should the ban prevent the use of digital bonuses entirely, or would it allow such bonuses only if they were offered consistently to all workers?
- Should such a law or regulation cover all digitalized variable pay practices across industries?
Notably, this is not a problem that U.S. employment law (in its current form) alone solves. If these workers for gig platform companies were classified as employees rather than independent contractors then they would be able to demand a wage floor, overtime compensation, and the right to organize a union. But given the low minimum wage and statutory carveouts for “waiting time,” Uber and Lyft as employers would still be able to use personalized pay to incentivize and control worker behavior.
Indeed, the core motivations of these companies to use algorithmic wage discrimination—labor control and wage uncertainty—could apply to many other forms of employment. Gig nurses, for example, could be offered different payments than their colleagues for the same work, at the same place, based on what the hiring platform knows about how much these nurses were willing to accept for previous assignments, or what they know about their debt and other financial obligations.
Or consider computer scientists laboring as employees for a company that uses software to monitor worker activity. They might have their pay manipulated according to the data extracted from their labor—in ways and for reasons that are invisible and unknown to them.
The United States boasts a clear legal tradition and social expectation of equal pay for equal work, which algorithmic wage-setting violates. Lawmakers and regulators need to examine the harms of these practices. There are still many details to figure out about how to implement a statutory or regulatory ban on algorithmic wage discrimination, but such a ban would remove some of the most egregious pay practices and be a step toward predictable, transparent, and fair pay.