When it comes to causality, no one technique should have all that power
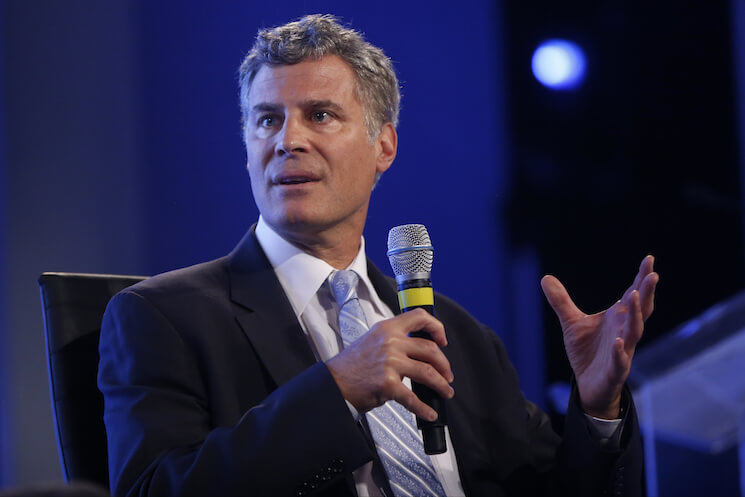
In the most recent interview in our “Equitable Growth in Conversation” series, our own Ben Zipperer talks with David Card of the University of California, Berkeley and Alan Krueger of Princeton University. The interview covers a number of areas, but it centers on Card and Krueger’s role in advancing empirical methods that help show causality. As you’ve probably heard a few hundred times in your life, correlation doesn’t imply causation. But the two economists sparked thinking about how other researchers could show the actual causal impact of a new policy or a shock to the economy—and this thinking is now a key part of the profession.
When it comes to showing causation in a hard science like physics or chemistry, the path forward is relatively well tread: Set up an experiment that controls for all the factors except the one you want to understand the impact of. But that’s not exactly possible when it comes to a social science like economics. You might want to understand what happens to economic growth when you implement a new policy, but it’s really hard to sort out the impact of everything else going on in the economy.
But researchers, like Card and Krueger, have figured out ways to tease out causality—one of which is a natural experiment. Think of Card and Krueger’s famous study on the minimum wage, for example. They used the fact that the minimum wage was going up in New Jersey but not Pennsylvania, and then looked at what happened to employment at fast food restaurants along the border in both states. The intent of raising the minimum wage wasn’t to cause an experiment, but the two economists used it as such.
Another way to get at causal effect is to use an instrumental variable. Let’s say you’re trying to understand how the amount of schooling a person has affects the wages he or she will earn. You’ll run into the problem that there are factors that affect how long a person will attend school while also affecting how much they might earn, such as innate talent. So how would you tease out just the effect of an exact year of school? You could find something that’s strongly correlated with the amount of time spent in school but not correlated at all with the other factors—say, talent—that might also affect wages, and then see how that affects how much a person earns.
A third option is to set up something very close to a real-world experiment, in a technique known as a randomized controlled trial, or RCT. A good example is the well-known Oregon Health Insurance Experiment. In 2008, the state of Oregon had enough money to expand its Medicaid plan but not enough money to expand it to all the people who wanted it. So the state ended up using a lottery to decide who got Medicaid and who didn’t. As the lottery was random, researchers could compare very similar people with and without health insurance knowing that difference was determined by nothing but chance. That certainty about randomness helps show the causal impact of health insurance.
But as Card says in the interview, all these methods have “some strengths and some weaknesses.” Natural experiments, for example, are great because they actually happen in the real world, but it’s not always clear how random they are. It’s tough to know how random the changes to policy are. At the same time, a randomized controlled trial is great for knowing the effect of the change in the trial to a great degree of confidence, but it’s hard to know how applicable those results are to circumstances outside those that happened during the trial. So while it might be nice to be able to point to one technique to rule over all others, giving such power to one technique probably isn’t for the best.